In a recent white paper titled Bi-Directional Deep Contextual Video Compression (DCVC-B), researchers Xihua Sheng, Li Li, Dong Liu, and Shiqi Wang proposed a new approach to enhancing B-frame compression for video encoding. The authors assert that their scheme “achieves an average reduction of 26.6% in BD-Rate compared to the reference software for H.265/HEVC under random access conditions” and even surpasses H.266/VVC on certain datasets.
The white paper positions DCVC-B as a new codec rather than an enhancement to existing ones. While it incorporates concepts from traditional codecs, its design is rooted in deep learning techniques. The proposed codec combines traditional video coding principles and advanced machine learning techniques. Its primary innovation lies in using bi-directional temporal contexts to enhance predictions and reduce redundancy. As described in the paper, DCVC-B employs “a bi-directional motion difference context propagation method for effective motion difference coding,” which substantially reduces the bit cost of motion vectors.
It also introduces a hierarchical quality structure during training, which assigns different quality coefficients across temporal layers, targeting optimal bit allocation within a group of pictures (GOP). The authors claim that these techniques enable the codec to leverage past and future reference frames more efficiently than its predecessors.
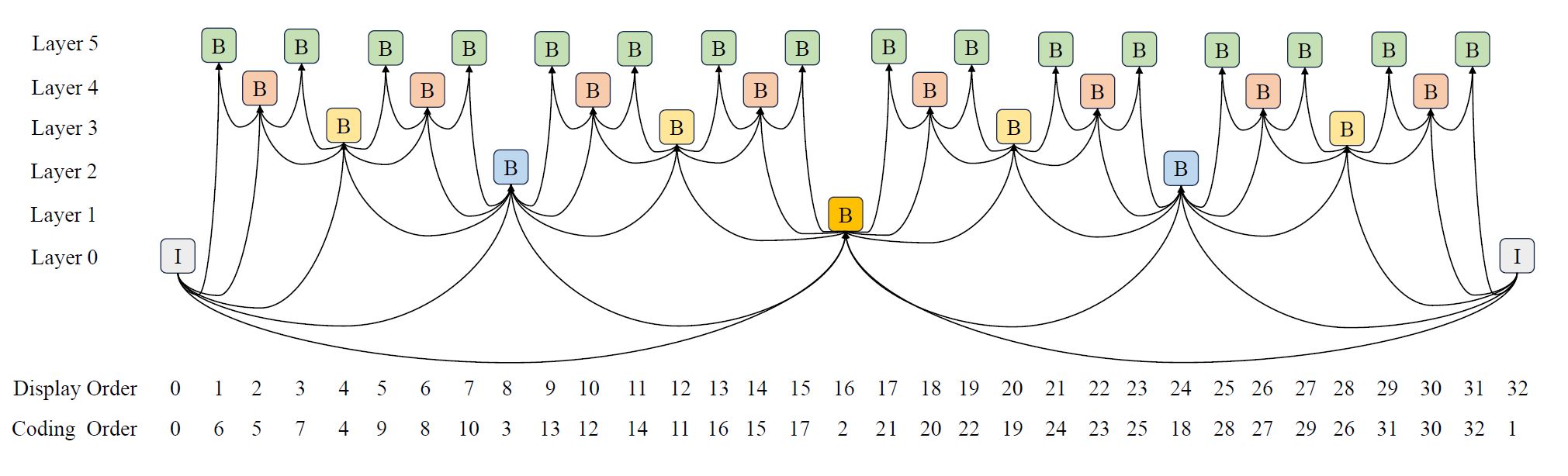
Training and implementation for DCVC-B involve complex workflows. The codec uses datasets such as Vimeo-90k for training and benchmarking. By incorporating pre-trained motion estimation models like SpyNet, the authors optimize the network to handle large GOPs and adapt to variable bitrates. The contextual encoder-decoder is trained with hierarchical quality settings, improving the model’s ability to allocate bits effectively across frames.
On the implementation side, encoding and decoding require significant computational resources, with experiments conducted on an NVIDIA 3090 GPU, a $900 card. While this demonstrates the model’s capability, it also highlights a key limitation in hardware requirements for practical deployment.
As reported in the paper, DCVC-B achieves compression performance improvements that range from a 15.8% to 42.9% reduction in BD-Rate over H.265/HEVC across different test scenarios as measured by PSNR and MS-SSIM.
Hardware requirements are a notable factor in its adoption. Unlike traditional codecs that can run reasonably efficiently on CPUs, DCVC-B relies heavily on GPUs for decoding. This dependency is driven by the need for neural network operations, such as motion compensation and entropy modeling, which are integral to the codec’s design.
Performance metrics provide a clear picture of DCVC-B’s standing. Encoding takes an average of 1.19 seconds per 1080p frame, while decoding takes 0.99 seconds, even with the GPU. This compares unfavorably to traditional codecs like H.266/VVC, where encoding and decoding can occur in real-time. However, the trade-off comes with compression gains; for example, DCVC-B achieves a BD-Rate reduction of 26.6% on average, outperforming many state-of-the-art alternatives, including other deep learning codecs such as DCVC-DC and B-CANF.\
Perspective
OK, it doesn’t beat VVC, but it’s intriguing to see how applying AI to a single compression parameter—B-frames—can yield significant results. By focusing on bi-directional temporal contexts and hierarchical quality structures, DCVC-B demonstrates the potential of AI to refine specific aspects of video encoding in ways traditional approaches haven’t fully explored. These methods could even be adapted to enhance traditional codecs like H.265/HEVC or H.266/VVC by improving the encoding process while maintaining a standards-compliant stream that existing decoders can handle.
While DCVC-B is unlikely to be the first major AI codec breakthrough, its techniques could contribute to future advancements. An AI codec incorporating similar innovations but with broader applications and optimized performance—or even hybridizing AI-driven encoding with traditional codecs—might be AI Codec 1.
The bottom line is that the researchers asked me—and they didn’t—I would advise them to focus on one of two paths: either create a codec designed for decoding on NPUs, like Deep Render, or apply AI techniques to improve the performance of existing codecs while maintaining compatibility with current decoders. Both approaches would help ensure their academic research sees the commercial light of day.